Unveiling the Power of Model Context Protocol (MCP) Servers in AI Agent Development
- Wrixit
- 4 days ago
- 9 min read
In the ever-evolving realm of artificial intelligence, the quest for more intelligent, adaptable, and efficient AI agents is relentless. At the forefront of this technological revolution is the Model Context Protocol (MCP) server, a transformative innovation that is redefining the landscape of AI agent development. As AI systems become increasingly integral to various industries, the need for a robust framework that enhances their interaction with dynamic environments has never been more critical. MCP servers address this need by providing a sophisticated protocol that enables AI models to seamlessly integrate with their operational contexts, thereby facilitating more intelligent and context-aware decision-making processes. This blog delves into the core functionalities of MCP servers, exploring their profound impact on AI agent development and the myriad benefits they offer to developers, businesses, and industries at large.
Understanding Model Context Protocol (MCP) Servers: Revolutionizing AI Agent Development
Model Context Protocol servers serve as a sophisticated framework designed to enhance the interaction between AI models and their operational environments. Traditionally, AI models have been developed in isolation, with limited consideration for the dynamic contexts in which they operate. This often led to inefficiencies and a lack of adaptability in real-world applications. MCP servers address this challenge by providing a structured protocol that allows AI models to seamlessly integrate with their surrounding environments, enabling more intelligent and context-aware decision-making processes.
One of the key features of MCP servers is their ability to facilitate real-time communication between AI models and external data sources. This capability is crucial for AI agents that need to operate in environments where conditions can change rapidly. By leveraging MCP servers, AI models can continuously update their understanding of the environment, allowing them to make more informed decisions. This real-time adaptability is particularly valuable in applications such as autonomous vehicles, where the ability to respond to changing road conditions and traffic patterns is critical for safety and efficiency.
Moreover, MCP servers play a pivotal role in enhancing the scalability of AI systems. As AI applications grow in complexity, the need for scalable solutions becomes increasingly important. MCP servers provide a modular architecture that allows developers to easily integrate new models and functionalities without disrupting existing systems. This modularity not only simplifies the development process but also ensures that AI systems can evolve and adapt to new challenges over time. By supporting seamless integration and scalability, MCP servers empower developers to build more robust and versatile AI agents.
Another significant advantage of MCP servers is their contribution to improving the interpretability and transparency of AI models. As the complexity of AI systems increases, understanding how these models make decisions becomes more challenging. MCP encourages the development of models that are not only accurate but also interpretable, allowing developers to gain insights into the decision-making processes of AI systems. This transparency is essential for building trust in AI technologies, particularly in critical applications such as healthcare and finance, where understanding the rationale behind AI decisions can have significant implications.
Furthermore, MCP servers facilitate collaboration and interoperability among different AI models and systems. In a world where AI applications are becoming increasingly interconnected, the ability to share information and insights across different platforms is invaluable. MCP servers enable this by providing standardized protocols for data exchange, allowing AI models to work together more effectively. This collaborative approach not only enhances the capabilities of individual AI agents but also fosters innovation by enabling the development of more complex and integrated AI solutions.
In summary, Model Context Protocol servers represent a significant advancement in the field of AI agent development. By providing a framework for real-time communication, scalability, interpretability, and collaboration, MCP servers are revolutionizing the way AI models interact with their environments. As AI continues to play an increasingly prominent role in our lives, the importance of MCP servers in enabling intelligent, adaptable, and trustworthy AI systems cannot be overstated.
Who Should Embrace Model Context Protocol (MCP) Servers in AI Agent Development?
The advent of Model Context Protocol (MCP) Servers marks a significant leap forward in the development of AI agents, offering unprecedented opportunities for innovation and efficiency. These servers are not just a technological advancement; they represent a paradigm shift in how AI agents are conceptualized, developed, and deployed. The stakeholders in MCP are diverse, ranging from AI researchers and developers to business leaders and policymakers, each playing a crucial role in the successful deployment and integration of AI technologies.
AI researchers and developers are at the forefront of MCP, as they are the architects of the models that drive AI systems. Their deep understanding of machine learning algorithms and data science is essential for creating robust and efficient models. By engaging with MCP, these professionals can focus on refining model architectures and improving performance metrics, leading to more accurate and reliable AI applications. The iterative nature of MCP allows researchers to experiment with different model configurations, fostering innovation and pushing the boundaries of what AI can achieve.
Business leaders and decision-makers also have a vested interest in MCP, as it directly impacts the strategic direction and competitive advantage of their organizations. By understanding and supporting MCP initiatives, executives can make informed decisions about resource allocation, investment in AI technologies, and the integration of AI into existing business processes. MCP provides a framework for aligning AI development with business objectives, ensuring that AI solutions are not only technically sound but also economically viable. This alignment is crucial for maximizing return on investment and achieving long-term business success.
Policymakers and regulators play a critical role in the MCP ecosystem. As AI technologies become increasingly pervasive, there is a growing need for regulatory frameworks that ensure ethical and responsible AI development. Policymakers who engage with MCP can better understand the technical and ethical implications of AI models, enabling them to craft policies that promote transparency, accountability, and fairness. By collaborating with AI researchers and industry leaders, regulators can develop guidelines that balance innovation with societal concerns, fostering an environment where AI can thrive while safeguarding public interests.
Educators and academic institutions focused on AI and machine learning are also prime candidates for adopting MCP Servers. By incorporating MCP principles into curricula, educators can prepare the next generation of AI professionals to navigate the complexities of model-centric development. This educational focus ensures that students are equipped with the skills and knowledge needed to contribute to the AI field effectively. Academic research can also benefit from MCP, as it provides a structured approach to exploring new AI methodologies and advancing the state of the art.
In summary, the successful implementation of MCP in AI development requires the active participation of a diverse range of stakeholders. Each group brings unique perspectives and expertise, contributing to a holistic approach that drives innovation, ensures ethical practices, and aligns AI technologies with broader societal goals. By fostering collaboration and understanding among these stakeholders, MCP can unlock the full potential of AI, paving the way for a future where intelligent systems enhance human capabilities and improve quality of life.
The Main Benefits of MCP and Its Importance in AI Development
The integration of Model-Centric Programming (MCP) into AI development has revolutionized the way artificial intelligence systems are designed, trained, and deployed. One of the primary benefits of MCP is its ability to streamline the development process by focusing on the model itself rather than the data. This shift in focus allows developers to create more robust and efficient AI systems by honing in on the architecture and algorithms that drive performance. By prioritizing the model, developers can ensure that the AI system is adaptable and scalable, which is crucial in a rapidly evolving technological landscape.
Another significant advantage of MCP is its contribution to improving the accuracy and reliability of AI models. By concentrating on the model's structure and parameters, developers can fine-tune the system to achieve optimal performance. This meticulous attention to the model's intricacies enables the creation of AI systems that are not only more precise but also more capable of handling complex tasks. As a result, MCP plays a pivotal role in enhancing the overall quality of AI applications, making them more dependable and effective in real-world scenarios.
MCP also fosters innovation by encouraging experimentation and iteration. In traditional data-centric approaches, the focus is often on acquiring and processing vast amounts of data, which can be time-consuming and resource-intensive. MCP, on the other hand, allows developers to experiment with different model architectures and algorithms without being bogged down by data constraints. This freedom to explore and iterate leads to the discovery of novel solutions and breakthroughs in AI technology. By promoting a culture of innovation, MCP helps drive the advancement of AI systems, pushing the boundaries of what is possible.
Furthermore, MCP enhances collaboration among AI developers and researchers. By emphasizing the model, MCP creates a common ground for professionals from diverse backgrounds to work together. This collaborative environment fosters the exchange of ideas and knowledge, leading to the development of more sophisticated and comprehensive AI systems. The shared focus on the model also facilitates the integration of interdisciplinary insights, which can lead to more holistic and effective AI solutions.
The importance of MCP in AI development is further underscored by its role in addressing ethical and societal concerns. As AI technologies become increasingly pervasive, there is a growing need for regulatory frameworks that ensure ethical and responsible AI development. Policymakers who engage with MCP can better understand the technical and ethical implications of AI models, enabling them to craft policies that promote transparency, accountability, and fairness. By collaborating with AI researchers and industry leaders, regulators can develop guidelines that balance innovation with societal concerns, fostering an environment where AI can thrive while safeguarding public interests.
In summary, the benefits of MCP in AI development are manifold, offering transformative advantages in the development of AI agents. From enhancing efficiency and collaboration to improving scalability and cost-effectiveness, MCP servers are revolutionizing the way AI systems are built and deployed. As the field of artificial intelligence continues to evolve, the role of MCP in shaping the future of AI will only grow, offering new possibilities and solutions to some of the most pressing challenges faced by industries today.
Getting Started with MCP in AI Development
Embarking on the journey of integrating Model-Centric Programming (MCP) into AI development can be both exciting and daunting. The first step is to understand the foundational principles that underpin MCP. This approach emphasizes the importance of the model itself, focusing on refining and optimizing it to achieve superior performance. Unlike traditional data-centric methods, MCP prioritizes the architecture, parameters, and algorithms that define the model's behavior. To get started, one must first ensure a solid grasp of the theoretical aspects of MCP, which involves studying various model architectures, understanding their strengths and weaknesses, and learning how to tailor them to specific tasks.
Once the theoretical groundwork is laid, the next step is to select the appropriate tools and frameworks that support MCP. Popular frameworks like TensorFlow, PyTorch, and Keras offer robust environments for developing and fine-tuning models. These platforms provide a plethora of pre-built models and libraries that can be leveraged to accelerate the development process. Familiarity with these tools is crucial, as they offer the flexibility to experiment with different model architectures and hyperparameters, which is a core aspect of MCP. Additionally, these frameworks often come with extensive documentation and community support, making it easier for developers to troubleshoot issues and share insights.
After setting up the development environment, the focus shifts to the iterative process of model refinement. This involves a cycle of training, evaluating, and adjusting the model to enhance its performance. Key to this process is the ability to effectively measure the model's performance using appropriate metrics. Understanding metrics such as accuracy, precision, recall, and F1 score is essential, as they provide insights into how well the model is performing and where improvements can be made. Moreover, the use of validation and test datasets is critical in this phase to ensure that the model generalizes well to unseen data, thereby avoiding overfitting.
Collaboration and continuous learning are also vital components of successfully implementing MCP. Engaging with the AI community through forums, conferences, and online courses can provide valuable insights and keep developers updated on the latest advancements in model-centric techniques. Sharing experiences and challenges with peers can lead to innovative solutions and foster a deeper understanding of MCP.
Finally, it's important to maintain a mindset of experimentation and adaptability. The field of AI is rapidly evolving, and what works today might not be the best solution tomorrow. Being open to new ideas and willing to pivot strategies when necessary is crucial for staying at the forefront of AI development. By embracing these principles and practices, developers can effectively harness the power of MCP to create more efficient, accurate, and robust AI models.
Conclusion: The Future of AI Development with MCP Servers
As we look towards the future, the Model Context Protocol (MCP) servers will undoubtedly play an increasingly vital role in the development and deployment of AI systems. Their ability to ensure the reliability, safety, and efficiency of AI models makes them an indispensable tool in the AI developer's toolkit. By providing a structured approach to verifying the correctness of AI models, enhancing transparency, and ensuring ethical compliance, MCP addresses many of the challenges associated with AI development. As AI continues to permeate various aspects of society, the importance of MCP in safeguarding the integrity and trustworthiness of these systems will only grow. Embracing MCP will be essential for advancing the capabilities of AI and ensuring its responsible and ethical deployment across various domains.
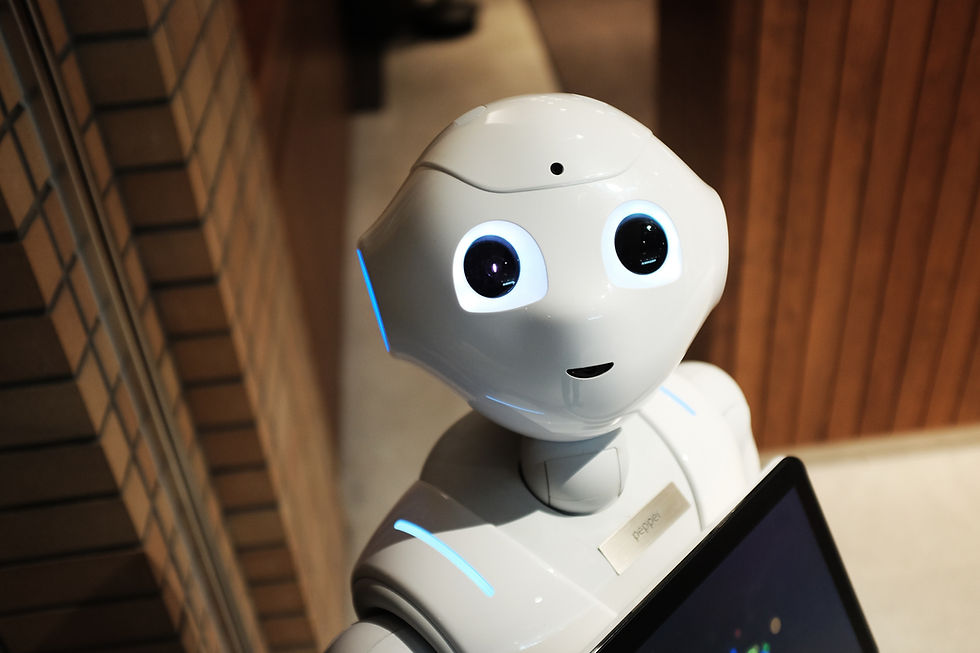
Comments